A recent academic paper proposes a five-step methodology for measuring how climate impacts to physical assets can inflict losses on financial institutions
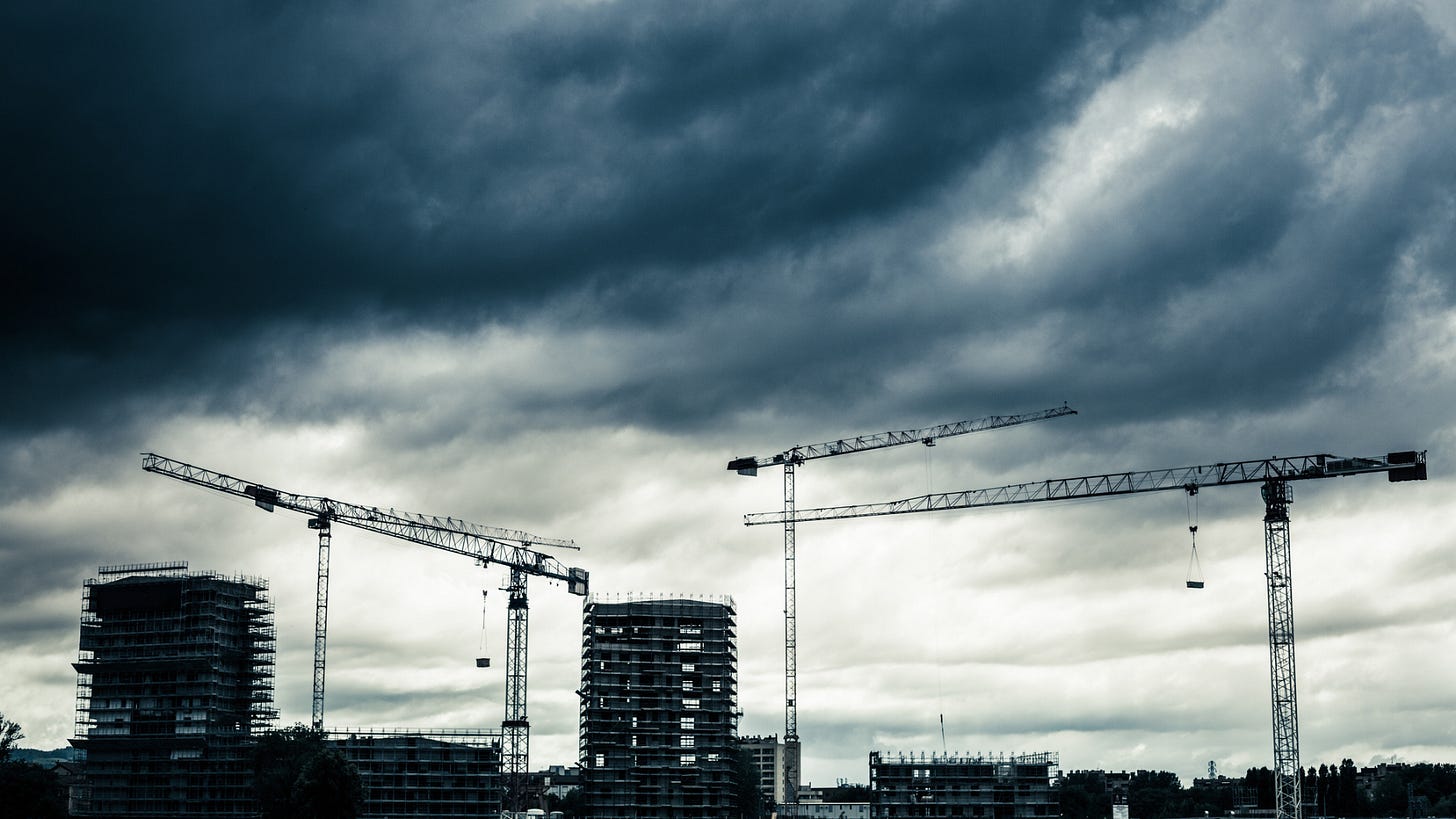
Climate physical risks are all over the headlines. On Monday, data released by the nonprofit First Street Foundation showed that half of all addresses in the lower 48 US states are exposed to wildfire risk, a percentage that will rise to 56% by 2052 as wildfires become more intense and frequent because of climate change. This followed research published by credit ratings agency S&P Global showing that physical risks could impact 4% of global GDP by 2050 without stronger emissions-reduction policies.
Such risk estimates look scary, but it’s hard to understand what they might mean for individual companies and the financial institutions that lend to and invest in them. There are four big reasons why, identified by a group of climate and finance academics in a must-read paper, “Asset-Level Climate and Cascading Financial Losses”: one, the lack of asset-level information on physical risks; two, the difficulty linking physical assets to their owners; three, the poor state of climate scenario analysis for physical risks; and four, the challenge translating climate impacts to physical assets into losses to financial assets. These factors stop banks and other institutions from painting a clear picture of their physical risk exposures.
The paper’s authors say financial institutions are especially vulnerable to “cascading risk” — the danger that climate losses incurred by a physical asset in one location ripple through a web of complicated financial relationships to hurt a financial institution in a completely different location, many miles away. The opacity of corporate ownership structures and poor data availability across asset classes makes this a largely hidden risk, too. An investor may have no idea that an equity listed in London could nosedive because of typhoons in Taiwan.
Right now, the financial industry and the climate service providers it relies on lack the tools to tackle cascading risk, the authors say. For starters, physical risk data is often “highly aggregated” and relies on “average sector values and approximate geo-locations.” This is a problem, since physical risk varies wildly by geolocation and type of asset. Even when such data is available in disaggregated form, such datasets are often black boxes, making it impossible for users to interpret or reproduce their outputs. Financial risk outputs based on these kinds of data are likely to be filled with errors and lead investors into bad decisions.
Financial institutions aren’t the only entities at risk of being misled on their physical risks because of poor data — regulators are, too. For example, for its recent economy-wide stress test the European Central Bank (ECB) gauged companies’ physical risks by evaluating the exposure of their corporate headquarters and subsidiary offices only. For want of data, they ignored companies’ factories, plants, distribution hubs, and data centers — arguably their most productive physical assets. This left the ECB with a skewed picture of the European Union’s physical risk exposure.
The paper’s authors offer a five-step methodological framework for conducting much more effective asset-level assessments of physical risk, which they tested on a sample of physical assets in Mexico, their beneficial owners, and the European financial institutions that invest in their stocks.
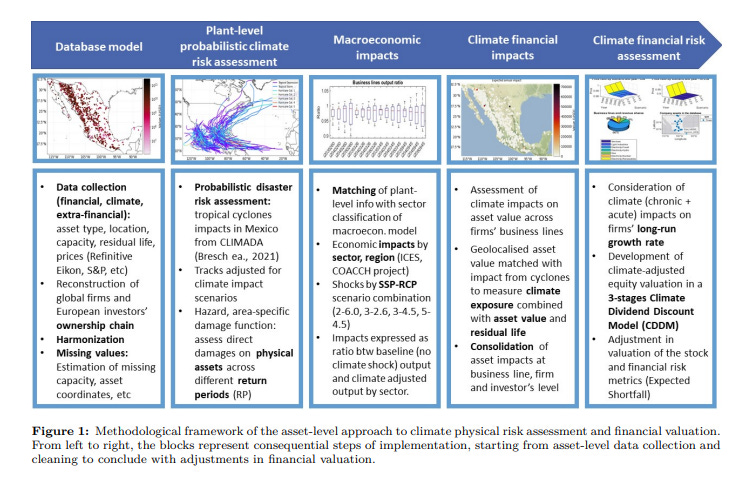
Source: Asset-Level Climate Physical Risk Assessment and Cascading Financial Losses
The first step is to build a database model “to collect and logically connect extra-financial, climate and financial information of individual assets and investors.” The key innovation proposed by the authors here is the breaking down of each individual company into “a portfolio of business lines and geographically distributed assets.” This makes it possible to identify those revenue-generating plants, factories, and other facilities that are most important to a parent company’s bottom line. In addition, disaggregating this way allows a company’s revenues to be mapped to specific economic sectors, which in turn enables an analysis of how sector-level physical risks may impact them.
The second step is to run a probabilistic climate physical risk assessment that can show the potential damage caused by particular acute physical risks — like tropical storms, floods, or wildfires — at the level of each physical asset. In the authors’ example, this assessment covers tropical cyclone hazards in Mexico over different timescales (2035, 2040, 2045, 2050) and different climate change trajectories.
The third step is to match these asset-level shocks with sector-level shocks from chronic, long-term physical risks. The authors calculate these latter shocks in their example using macroeconomic models that project future economic conditions based on select climate scenarios.
The fourth step is to determine how these combined shocks change the financial valuation of each physical asset and how, in turn, these impacts manifest at the business line, firm, and investor levels.
Finally, the fifth step is to translate these shocks into adjustments to the valuation of financial assets linked to the owners of the physical assets. In the paper, the authors use a homebrewed “Climate Dividend Discount Model” to calculate changes to the stock prices of the companies in their sample.
Applying this methodology to the sample of Mexican assets and beneficial owners produced some interesting results. The authors found that Expected Annual Impacts (EAI), meaning impacts weighted by the frequency of physical risk events, manifested as a -1.29% change in stock prices on average. This looks small, but it masks the much greater size of the worst-case loss. One stock was projected to lose -13.07% following a 1-in-250 year tropical cyclone event. Of course, what today counts as a 1-in-250 year event may occur far more frequently in the near future because of the nonstationarity of climatic conditions.
The authors also estimated the risks to a hypothetical portfolio invested in all the sampled companies. For this, they used expected shortfall (ES) — a metric showing the expected return on a portfolio in the worst of cases. They calculated a -4.02% ES under EAI, and a -12.48% ES under the 1-in-250 year scenario. That’s the kind of potential loss that should make risk managers and financial supervisors sit up straight, especially since the authors’ assessment covered only one type of climate peril in one country.
But while the five-step framework is certainly comprehensive, it is not without problems. For one, as the authors admit, building the database model in the first step is labor intensive. In order to disaggregate a company into “a portfolio of business lines and geographically distributed assets,” it is necessary to split apart total revenues using information on business units, product types, and their respective sales and prices. While the authors said machine learning techniques can help speed the process, they admitted that a “manual reclassification step is inevitable.” This suggests the approach could be too burdensome for institutions and regulators to replicate at scale.
Then there’s the enduring issue of data gaps. Using multiple asset-level databases, the authors were able to identify 3,493 energy-intensive physical assets in Mexico that they could match to beneficial owners, of which 1,820 could be linked to companies invested in by 1,014 European financial institutions. That’s a lot of assets and a lot of institutions, but it’s unclear from the paper how many assets the authors were unable to link to beneficial owners or their end investors. Even for those physical assets they could identify, the authors had to use a “preprocessing pipeline” to estimate missing values — like production capacity and residual life. This was necessary to scrub the list of irrelevant entries, but it also populated the resulting database with synthetic values that may have under- or over-estimated the worth of certain assets.
Fixing this problem is the focus of the emerging field of spatial finance — the marriage of geospatial data and analysis into financial theory and practice. What would also help are more fulsome physical asset disclosures by companies themselves. This is what regulatory initiatives like the US Securities and Exchange Commission’s (SEC) proposed climate risk disclosure rule are trying to achieve. Indeed, the SEC’s proposal would require companies to identify the “location of the properties, processes, or operations” subject to identified physical risks at the ZIP code level. Such disclosures would greatly enhance the supply of geo-localized asset data.
The barriers to effective asset-level physical risk assessments are not insurmountable, and are likely to shrink in the face of technological advances and new regulations. Getting financial institutions to establish sound methodologies to conduct these assessments is another challenge entirely. The paper discussed here offers one way forward. Climate risk managers would be wise to heed its lessons.